
Artistic ollustration of InClinico, an AI tool for predicting clinical trial outcomes
Roughly nine out of ten clinical trials fail as a result of factors such as lack of efficacy and unmanageable toxicity, as the journal Pharma Excipients has noted.
After seven years of development, Insilico Medicine has scored a breakthrough with its generative AI tool, inClinico. In particular, the tool demonstrated 79% accuracy in predicting the outcomes of real-world phase 2 and 3 trials.
While predicting clinical trial performance has been a sort of Holy Grail in drug development, traditionally, it has been elusive. Several factors have constrained adoption: limited peer-reviewed evidence backing the use of ML models, a “black box” dilemma making clinicians wary of ML predictions, logistical hurdles in integrating ML into clinical workflows and EHR systems and a need for ample high-quality training data. Also, regulatory concerns over ML models for trials and philosophical qualms about favoring ML over human judgment act as barriers.
InSilico is thus believes the accuracy of InClinico in predicting clinical trial outcomes could be a game-changer in drug development.
The company also recently announced that one of its AI-discovered drugs has moved to phase 2.
How InClinico can predict clinical trial outcomes
Incorporating transformer-based AI models, the AI predictive tool inClinico is based on a collection of models using generative AI and multimodal data sources including text, omics, trial design and drug properties. The developers of tool trained it on more than 55,600 phase 2 clinical trials.
The peer-reviewed journal Clinical Pharmacology and Therapeutics journal published the research. InSilico also notes that pharmaceutical companies and financial institutions have validated InClinical through retrospective, quasi-prospective and prospective validation studies.
The platform achieved a score of 0.88 on the Receiver Operating Characteristic Area Under the Curve (ROC AUC) in predicting the transition from phase 2 to 3. ROC AUC stands is a statistical measure used in machine learning and data analytics to gauge the performance of a binary classifier system. AUC values lie between 0 and 1. Higher values indicate that the model is better at predicting 0s as 0s and 1s as 1s. An AUC value of 0.5 indicates that the model fares no better than random chance.
Insilico touted the tool’s usefulness to investors, stating that it supported a 35% 9-month return on investment in a virtual trading portfolio.
InSilico CEO dishes on the power of the AI predictive tool in clinical trials
To learn more about InClinico, we reached out to Alex Zhavoronkov, founder and CEO and co-author on the paper.
How does Insilico’s generative AI, inClinico, use multimodal data to predict the outcome of clinical trials?
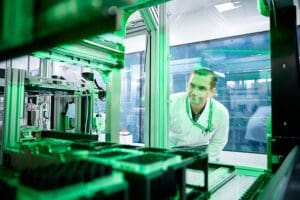
Alex Zhavoronkov in an AI-powered autonomous robotics lab called Life Star in Suzhou that performs target discovery, compound screening, precision medicine development and translational research. [Insilico Medicine]
Using machine learning and AI, we built models based on data points specific to successfully launched and failed drugs, including clinical trial design, biological features of the disease, drug targets and patient criteria. We combined these models into our AI tool. For every evaluated phase 2, inClinico generates a probability of success for proceeding into phase 3.
We have been training inClinico on clinical trials, drugs and diseases since 2014 and as the technology has advanced into multi-modal large language models and other generative AI technologies, we’ve been able to improve its predictive capabilities so that it can serve as a tool to help companies direct their research dollars and expertise toward programs that have the best likelihood of success and to capture and utilize valuable information about the programs that have failed.
The press release describes an accuracy of 79% in predicting the outcomes of real-world trials. How did the company measure this benchmark?
Zhavoronkov: Our researchers collected phase 2 clinical trial data from 55,653 phase 2 trials from clinicaltrials.gov, which come from a variety of public sources, including pharma press releases and publications. The data was curated by both biomedical experts and by a generative large language model. Our AI tool then mapped these trials to drugs and diseases using a natural language processing pipeline based on the state-of-the-art Drug and Disease Interpretation Learning with Biomedical Entity Representation Transformer (DILBERT). Using this data, we built a model for predicting the phase 2 clinical trial probability of success which is defined as the transition of drug-condition pair from phase 2 to phase 3.
Validation studies for this paper were based on 17 clinical trials whose phase 2 outcomes were made public by August 2022. Based on these studies, inClinico’s predictive ability was found to be 79% accurate. We determined a phase 2 clinical trial as “successful” based on three factors: the statistical and clinical significance of efficacy and safety endpoints; a company’s decision to transition the drug program to phase 3 and the momentary increase of company’s stock price in response to clinical trial results.
As noted in the paper: “While Machine learning (ML) and artificial intelligence (AI) are widely used in clinical trial operations by pharmaceutical companies, there have been no validated reports on the implementation of ML/AI predicted probability of technical and regulatory success (PTRS) systems within pharma research and development (R&D) processes.”
Could you elaborate on the findings that “target choice” has more impact on clinical trial outcome prediction than “trial design”?
Zhavoronkov: The traditional method of finding targets in the pharma industry is a combination of academic research and serendipity. But we know that the vast majority of these hypotheses will ultimately fail when drugs reach clinical trials, suggesting that the complexity of diseases and biological mechanisms makes finding precise targets for disease incredibly difficult.
AI can help us find those critical connections, analyzing massive amounts of diverse data – omics data, grants, clinical trials, compounds and publications – in the context of a disease of interest in order to produce a ranked list of potential targets. That is how our AI target discovery engine PandaOmics works, to not only efficiently, but quickly, identify promising targets for disease – and it has identified targets for 30-plus drugs that we are developing, including three in clinical trials. AI not only has a huge role to play in helping us find new promising targets, but also in designing new molecules to fit these targets and predicting the likelihood of success in bringing them through clinical trials.
What’s next for InClinico? What further advances do you expect in terms of prediction accuracy?
Zhavoronkov: Ultimately, I would like this tool to be deployed broadly as with broader use, it will further improve. We utilize an approach we call Reinforcement Learning from Expert Feedback (RLEF) – the more expert feedback we receive from analysts using the tool for making predictions, the better it becomes. At this time, we can only predict small molecule first-in class single-agent targeted therapeutics. We estimate about 20–25% of the trials to be predictable with our current tool with meaningful accuracy. I hope that with our new laboratory robotics capabilities, we might be able to expand into predicting success rates for combination therapies and help select the best combination for targeted therapies.
Filed Under: clinical trials, Data science, Drug Discovery, Drug Discovery and Development, machine learning and AI, Regulatory affairs