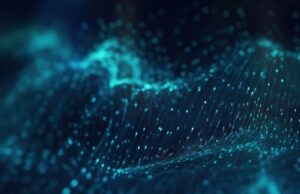
[Adobe Stock]
The initiative has the firm support of the company’s CEO Jan G. J. van de Winkel, who expects AI to help enhance R&D efficiency in the coming years. Already, the company has succeeded in winning FDA approval for the antibody epcoritamab in lymphoma within five years after the first patient was dosed. The approval timeline was “a world record,” van de Winkel told Medwatch, adding that the company believes its investments in data science will unlock further breakthroughs. “AI and access to digital information will play a big role in this,” he noted.
Genmab prioritizes being a data-driven firm that bridges the gap between research scientists and data scientists while democratizing data access across the organization. The company’s data strategy “started with the premise that we have to begin with data, and we have to start with the people who know how to interrogate that data,” Hamadeh said. Leadership made strategic investments in analytics, AI, and cloud infrastructure to support this cultural shift. As Hamadeh says, “we strive to do a few things: ask what is the data relevant for this practice of data science and AI in this vertical within the company, and try to marry the people with data science expertise side by side.” By embedding data scientists within business functions, Genmab has fostered “a culture of experimentation and evidence-based thinking.”
Building out an AI toolkit
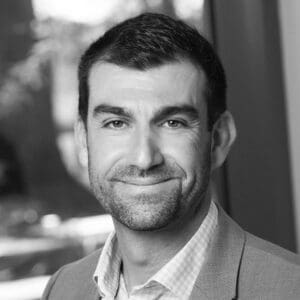
Hisham Hamadeh, PhD
Genmab uses a diverse range of AI technologies. “We’re not married to any one approach,” Hamadeh said. Depending on the use case, it might employ an unsupervised machine learning model for discovery. In other cases, where a specific hypothesis is involved, it could use supervised machine learning to train a model for a specific function. “We are also advanced in our use of large language models (LLMs) to accelerate tasks such as document generation and analytics using agents,” Hamadeh added. Agents are “fascinating tools,” he said, capable of orchestrating tasks such as fetching scripts in Python, reading datasets, plotting datasets, and making inferences. Additionally, Genmab uses computer vision for myriad applications ranging from in vitro imaging of cells to analyzing histology images. “Most exciting recently is the application of AI to radiology scans, predicting trial outcomes or informing patient responses,” Hamadeh noted. “We always pick the right models and approaches based on what we’re trying to accomplish with the data and the problem at hand.”
How Genmab sizes up the strengths and weaknesses of various text AI technologies
The company has explored large language models (LLMs) for regulatory document generation, tapping a range of techniques including zero-shot, one-shot, and two-shot approaches. These methods, along with advanced strategies like fine-tuning or retrieval-augmented generation (RAG) and natural language processing, a mainstay of automated document generation, provide comes in handy for more structured tasks. “Sometimes you don’t want to use a big sledgehammer when you could use something lighter to generate a document where you know exactly where everything goes,” Hamadeh said.
LLMs come in handy when inference is needed, “adding context that a human being usually does when they summarize content from multiple streams of evidence,” Hamadeh said. The company is exploring such strategies to help with the generation of documents related to clinical development and regulatory submissions.
While off-the-shelf tools could, say, suggest a first draft for a job description, they can stumble when the context requires more specific technical details or precise formatting. “This is where one could play with fine-tuning or RAGs to achieve something that is closer to what you need,” Hamadeh said.
Staying grounded amid the dizzying pace of AI progress

FDA granted accelerated approval to epcoritamab-bysp (Epkinly, Genmab) on May 19, 2023 for relapsed or refractory diffuse large B-cell lymphoma (DLBCL) not otherwise specified, including DLBCL arising from indolent lymphoma, and high-grade B-cell lymphoma after two or more lines of systemic therapy. AbbVie is also involved in commercializing the antibody.
The explosion of AI tools is something of a “double-edged sword,” Hamadeh reflected, noting the challenge of keeping up with the continuous drumbeat of AI advances. “It can be exciting, but also very distracting,” he said. Even an expert in the field such as a data scientist or AI practitioner has “their work cut out for them because there’s so much to learn,” he added. “Some of these LLMs are fairly new and have accelerated like a hockey stick.” Studying the strengths and weaknesses of the latest models, and how to derive value from them, can lead practitioners into some “very deep rabbit holes,” Hamadeh said. This fact requires a disciplined approach to balance staying abreast of the most recent advances while remaining grounded in business realities.
To build or buy? Factors in selecting AI tools
The variety of AI tools and approaches also plays into the calculus of whether Genmab builds or buys a given technological tool in its toolbox. “Directionally, you never want to recreate something that’s available externally, because not only is there the cost to build something, but also the cost of maintaining what you’ve built,” he said. Given its prime objective of bringing life-saving medicines to patients, developing software is a means to an end.
The company tries to reserve custom software development for bespoke needs, “maybe writing code or creating different applications for something very specific that we believe has no equivalent,” Hamadeh said. “We always push the envelope on whether a solution exists and, if it does, how much customization it requires.”
In addition to cost, factors that weigh into its decision-making include timeliness of access and repeatability and scalability. “If we have 50 types of documents for AI to generate, are we going to negotiate with 50 different vendors, or do we seek a solution platform, whether built internally or acquired externally?” he asked. “We also look at efficiencies and scale.”
On the AI advantages of being a mid-sized company
Hamadeh credits Genmab’s size as a mid-sized company as providing significant advantages when it comes to integrating data science across the organization. “This adaptability is something more feasible in a smaller organization than in a larger one — partly due to the ability to adopt and implement new ways of doing things in a more nimble fashion,” he said.
“Employees gain portable skills that transfer as needs evolve,” he said. “If a job changes, you can adapt, applying what you’ve learned in another area. In addition, the ecosystem of data science and AI in a mid-sized company allows for much transferable learnings from various business functions and applications that can accelerate the development of solutions.”
Ultimately, data science proficiency is essential. “You need not only technical skills but also functional expertise,” Hamadeh said.
“From a data science perspective, this concept of algorithmic leadership is vital. People should be accustomed to not only leading people but also algorithms. The only way to scale a data science practice is if everyone becomes a citizen data scientist. With today’s tools, like LLMs, that aim is becoming even more attainable. Never in history have these sophisticated tools been at the fingertips of literally everyone within an organization. That is something we continue to strive for, scaling our organization in data science and AI.”
Filed Under: Data science, Drug Discovery and Development, machine learning and AI