
[yoshitaka/Adobe Stock]
Yet for organizations to fully realize AI’s promise is not simple. The task requires oversight, transparency and diverse collaboration. Core considerations include educating users to build trust in AI tools and ensuring the clinical precision of medical-grade AI algorithms. From unraveling the ‘black box’ of algorithms to safeguarding patient privacy, this article provides a checklist to help organizations responsibly incorporate AI into clinical trials.
1. Educate users to build confidence in AI technology

This line graph shows the annual count of research mentions for ‘Artificial Intelligence’ and ‘Clinical Trials’ on PubMed from 2017 to 2022. A sizable increase in mentions occurred from 2017 to 2020, followed by a slight decrease in 2021 and 2022.
When introducing new AI tools, it is vital to educate various stakeholders to ensure they understand how the technology works. “The better we are able to educate the user, the more confident they will be in the product,” said Dr. Todd Rudo, chief medical officer at Clario. Clear communication about AI training and validation can overcome resistance. If stakeholders, whether patients, providers or pharma companies, understand what went into the development of an algorithm, Rudo said, their views on the technology will evolve in turn.
Another strategy to win support from medical professionals is to design AI-systems with an evidence-based approach. A study by Qian Yang, assistant professor of information science at Cornell, supports that conclusion. Yiang created a clinical decision support tool that mimics doctor-to-doctor consultations. She found that doctors respond more positively to AI tools that act like colleagues, providing relevant biomedical research to support decisions.
2. Unravel the ‘black box’ through responsible algorithm development and deployment
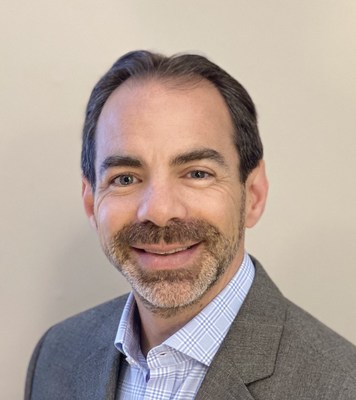
Dr. Todd Rudo, chief medical officer of Clario
In AI, the “black box problem” refers to the quandary where only the inputs and outputs of a model or system are clear. That is, data goes in and data comes out, but the intermediary step is opaque. In medicine, the problem has stoked tension between ethicists, programmers and clinicians, as BMJ has noted.
While concerns around AI’s “black box” nature persist, training and controlled deployment can mitigate many headaches related to it. “If you build the algorithm responsibly by understanding the data set that you’ve used to train and validate, this ‘black box’ concern goes away because you’ve proven it gives the right answer,” Rudo said. Maintaining algorithm integrity from development through deployment can further build confidence.
3. Implement AI for real-time quality checks in data collection
AI techniques can monitor and provide instant feedback on clinical trial data quality. Rudo illustrates this point with an algorithm that can instantly review ECG data and give real-time feedback to the site. Such an algorithm can indicate which ECGs were quality recordings and which weren’t.
In addition, real-time AI quality checks can enhance the progression of a study by pinpointing potential problem areas or opportunities for improvement. “With future cohorts, you have the opportunity to improve quality as the study goes on,” Rudo said. While humans can take the lead on work like this, it tends to be time-consuming and much slower than with AI. Rudo highlighted the potential of AI to promote clinical trial efficiency by enabling rapid turnaround feedback to sites.
4. Secure patient privacy in medical images and data
AI tools can automatically detect and obscure identifiable patient information in medical images to safeguard patient privacy. A number of medical scans such as sinus CT scans, for instance, have associated renderings with patient-identifiable information. “We have an AI-enabled tool that’s able to essentially deface that image — blurting it out automatically — before anyone has even viewed it,” Rudo said.
In terms of other types of medical data, techniques like federated learning can protect patient privacy by allowing the training of AI models with data from multiple institutions while keeping sensitive patient information securely within the confines of each institution involved in the research. Such practices align with regulations such as Health Insurance Portability and Accountability Act (HIPAA) and FDA guidelines, which specify robust safeguards for patient privacy such as data anonymization and tight access control for sensitive data.
5. Reduce noise and enhance efficacy through AI
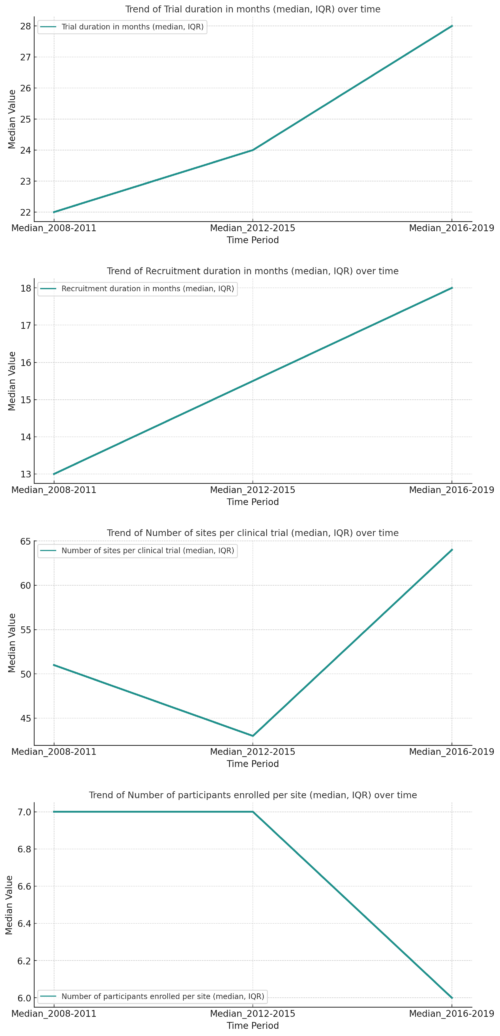
Trends in recruitment performance metrics for industry-sponsored phase 3 clinical trials conducted globally from 2008 to 2019. Deteriorating clinical trial metrics from 2008 to 2019 underscore the need for AI techniques to bolster efficiency. The visualizations highlight the eroding performance in terms of the median values for trial duration, recruitment duration and number of participants enrolled per site. Data from PLOS ONE. Distributed under the terms of the Creative Commons Attribution License.
Inconsistent or imprecise data can obscure the clinical trial results, hindering the ability to detect clinical success. “If you have very noisy data, meaning not precise data, it can be difficult to detect [clinical] success,” Rudo said. This lack of precision can hide a genuine signal of a drug’s efficacy within the surrounding noise.
False positives can similarly mislead investigators. AI techniques, however, can help ensure data quality. “When it comes to safety, data quality is important because you can have false positive safety signals that lead to a drug being sort of falsely accused, if you will, of having a side effect that it does not,” Rudo said. Such false positives could lead a sponsor to give up on developing a drug when it is prematurely warranted, underscoring the value of AI techniques that can support quality.
6. Explore AI applications to enhance medical imaging
Numerous AI techniques can improve imaging workflow, quality, analysis and interpretation. Clario alone is using dozens of AI-enabled applications with many related to medical imaging quality. “Data quality ultimately is critical for clinical trial results,” Rudo stressed.
In addition to Clario, numerous other companies and research groups are focused on using AI to enhance medical imaging contexts. For example, Microsoft and Nvidia are working together to explore how Nuance’s Precision Imaging Network (PIN) cloud platform can be combined with Nvidia’s open-source MONAI framework for developing medical imaging AI models. Their collaboration aims to foster clinical integration and scalability of AI-driven medical imaging analysis.
7. Balancing human oversight with AI in clinical trials: Adopt a ‘trust but verify’ philosophy
The phrase “trust but verify,” a Russian proverb, gained international prominence after the Russian historian Suzanne Massie taught it to then-U.S. President Ronald Reagan. Reagan used the phrase several times in the context of nuclear disarmament discussions with the Soviet Union. Eventually, the proverb became one of Reagan’s signature phrases.
The phrase is equally valid in the realm of clinical trials. While AI can aid clinical trial analysis, human oversight remains irreplaceable at least for the foreseeable future. “I don’t think we’re going towards fully AI-run clinical trials,” Rudo stressed. Instead, humans should scrutinize AI-enabled conclusions, adopting a “trust but verify” approach.
8. Mitigate algorithm bias through scientific validation
To prevent bias and ensure scientific integrity in AI applications within clinical trials, rigorous training of algorithms is vital. Here, machine learning systems require sufficient validation data to ensure accuracy after training. “Let’s say you have a million data points,” Rudo said. You might use 600,000 of them to train the algorithm. “And now, you still have 400,000 left that you can use to validate your work,”
9. Foster cross-functional collaboration
AI development in a clinical trials context requires a unique blend of technical and scientific expertise. “Obviously at the core of any AI program is the software people — the people who actually understand the programming,” Rudo said. But scientific insight is also essential. “One of the really critical points is having the scientific expertise to know how to best apply AI tools,” Rudo said.
Experts play a vital role in interpreting and contextualizing the data that algorithms analyze, and can help identify and mitigate potential biases in AI algorithms.
“Potential algorithmic bias is a concern that requires scientific expertise to identify and mitigate by critically reviewing the AI development process and validation data,” Rudo said. Experts also guide the responsible and accurate use of AI tools in a clinical trial context. “One of the things that’s critical in a cross-functional team is making sure that our developers are using the best available training datasets,” Rudo noted.
Filed Under: clinical trials, Data science, Drug Discovery, Industry 4.0, machine learning and AI