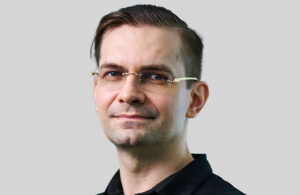
Alex Zhavoronkov, Ph.D.
Idiopathic Pulmonary Fibrosis (IPF), a devastating lung disease affecting millions with increasing incidence, may have a new treatment hope thanks to a novel inhibitor of TNIK, a kinase newly implicated in fibrosis, identified using generative AI drug discovery platforms in just 18 months.
Researchers at Insilico Medicine, along with international collaborators, harnessed the power of AI platforms PandaOmics and Chemistry42 to make a breakthrough discovery. Nature Biotechnology recently published the group’s findings, showcase the potential of AI to accelerate drug discovery.
The scientists identified TNIK as a promising therapeutic target for fibrotic diseases and developed INS018_055, a potent TNIK inhibitor. Preclinical models demonstrated INS018_055’s superior anti-fibrotic and anti-inflammatory effects compared to current treatments.
Beyond fibrotic disease
TNIK holds potential beyond fibrotic diseases. It’s implicated in aging, cancer and weight loss without muscle wasting. Recent animal studies demonstrate TNIK’s crucial role in regulating metabolism, suggesting it could be a target for new weight loss drugs that reduce fat mass without harming muscle tissue. Insilico Medicine’s AI-driven research further points to TNIK’s involvement in key hallmarks of aging, including altered intercellular communication, stem cell exhaustion and genomic instability. Additionally, a number of studies point to the promise of TNIK inhibition as a treatment for cancers like colorectal and thyroid cancer.
Check out the video below that animated graphic accompanying the paper publication:
To accompany the research, Insilico Medicine also released a custom GPT users can use to explore the paper. The company continues be among the most vocal in disseminating the benefits of AI in drug discovery.
To learn more about its recent developments, we caught up with Alex Zhavoronkov, Ph.D., founder and CEO of Insilico Medicine over email. In the following interview, he discusses the significance of the AI-driven discovery of TNIK as a novel target for fibrotic diseases and the development of INS018_055 as a potential treatment for IPF. He highlights the transformative potential of AI in accelerating drug discovery and improving patient outcomes. Zhavoronkov also shares his vision for the future of AI-driven drug discovery and its application across various therapeutic areas.
Could you say more on a pivotal decision-making moment where PandaOmics’ multi-omics data critically influenced the prioritization of TNIK over other potential targets?
Zhavoronkov: We wanted to identify a target that was highly implicated in both diseases and the aging process, and fibrosis is a major pathological process associated with the hallmarks of aging. We applied our PandaOmics target-discovery platform to mine lung fibrosis datasets. Considering druggability and novelty, protein and receptor kinases were set up with high priority in fibrosis datasets analysis.
Regardless of the lack of proof of concept of a TNIK inhibitor targeting IPF, we made the decision to pursue TNIK for drug discovery based on this AI analysis.
Target ID scoring was calculated on 15 IPF datasets collected from the GEO database. (datasets used include: GSE93606, GSE38958, GSE28042 and GSE33566 derived from blood tissue and GSE101286, GSE72073, GSE150910, GSE92592, GSE52463, GSE83717, GSE21369, GSE15197, GSE99621, GSE138283 and GSE24206 derived from lung tissue).
In contrasting TNIK with specific drugs like nintedanib and pirfenidone, what unique mechanistic actions or patient outcomes does a TNIK inhibitor potentially offer?
Zhavoronkov: TNIK inhibitor INS018_055 has been profiled extensively in preclinical studies in terms of mechanism and safety. In brief, INS018_055 suppresses a few key profibrotic signaling pathways including TGF-β, Wnt/β-catenin and YAP/TAZ. In assays performed in primary cells derived from IPF donors, INS018_055 showed more potent anti-EMT and anti-FMT effects than nintedanib. (Full data in the paper’s supplementary information). In preclinical models, INS018_055 showed both anti-fibrotic and anti-inflammatory activities. The combination of INS018_055 with pirfenidone [Genentech-developed drug that was FDA approved in 2014] showed improved efficacy in the animal model.
With a favorable safety profile in preclinical studies, we expect TNIK inhibitor INS018_055 to offer better safety and benefit to patients and serve as an option for patients who failed to improve from the current antifibrotic treatment.
How did you determine TNIK’s structure? Did this insight directly influence INS018_055’s design and your confidence in its binding?
Zhavoronkov: We started the design process with the available crystal structures of the TNIK kinase, and looked to the established strategy of targeting kinases by selecting the ATP-binding site. Our generative AI engine Chemistry42 was directed to make small molecules that could form hydrogen bonds with the Cys108-NH of the TNIK hinge region. We further optimized these molecules by targeting adjacent allosteric pockets in addition to the active site.
We then selected the AI-designed structures that demonstrated the best synthetic accessibility, novelty and medicinal chemistry properties, synthesizing and testing less than 80 to arrive at our lead compound.
Can you detail the ADME properties of INS018_055 that underscore its clinical candidacy?
Zhavoronkov: In the supplementary data of this paper, we optimized some ADME properties, such as clearance in human and mice liver microsomes, CYP inhibition and kinetic solubility. And the phase 0 and 1 trials exhibit favorable PK profiles.
Among other TNIK inhibitors, NCB-0846 is one of the most studied. The majority of the studies focus on its anti-cancer effect. NCB-0846 at low concentration (0.1 μM) demonstrates inhibitory effects on some other kinases including cyclin-dependent kinase (CDK)2, and it was also reported to impair cell viability, while INS018_055, as we found in multiple in vitro studies, has high CC50 values, which mean it only impairs cell viability in high concentrations.
Considering the different kinase profile of INS018_055 compared to NCB-0846, and the low cytotoxicity and favorable preclinical safety data of INS018_055, pharmacokinetic (PK) or toxicokinetics (TK) head-to-head comparisons between INS018_055 and NCB-0846 were not performed.
What are the primary efficacy and safety biomarkers for INS018_055 in upcoming Phase 2 studies, and how do they correlate with its mechanism of action?
Zhavoronkov: Two phase 2a clinical trials of INS018_055 for the treatment of IPF are being conducted in parallel in the US and China. They are both randomized, double-blind, placebo-controlled trials aiming to evaluate the safety, tolerability, pharmacokinetics and preliminary efficacy of INS018_055. Treatment-emergent and treatment-related adverse events will be evaluated based on clinical assessments. Lung function in IPF patients is to be evaluated as the primary efficacy parameter. Several cytokines correlating with IPF progression, inflammation and Wnt/β-catenin will be evaluated as exploratory PD markers for target engagement assessment. No specific biomarkers were used for patient stratification in these two trials.
How readily adaptable are these AI platforms to other therapeutic areas?
Zhavoronkov: This lead drug is a testament to our AI drug discovery platform and capabilities, but it is hardly alone. Since 2021, Insilico has nominated 18 preclinical candidates – and we have a comprehensive portfolio of over 30 assets, including six programs that have advanced to the clinical stage. The majority of our AI-designed drugs are in cancer (including a MAT2A, ENPP1, DGKA, KAT6 and USP1 inhibitor) and we are also advancing potential treatments for kidney fibrosis, IBD and COVID-19. We have also partnered a number of these assets, signing major global out-licensing deals with Sanofi, Exelixis and Menarini.
Filed Under: clinical trials, Drug Discovery, machine learning and AI, Pulmonology, Rare disease