
Insilico’s AI-driven drug discovery process, showcased in their AI-powered robotics lab [Image courtesy of Insilico Medicine]
In an interview, Alex Zhavoronkov, founder and CEO of Insilico Medicine, shared that Phase 1 trials of INS018_055 have been completed in New Zealand and China, showing a favorable safety profile. Insilico plans to launch Phase 2 trials in 2023 to further evaluate the drug’s efficacy and tolerability. If successful, INS018_055 could provide a new treatment option for patients with idiopathic pulmonary fibrosis. The trial results also demonstrate the potential of AI to accelerate drug discovery and development.
When was INS018_055 first nominated as a preclinical candidate for the treatment of IPF and when was it advanced to clinical validation?
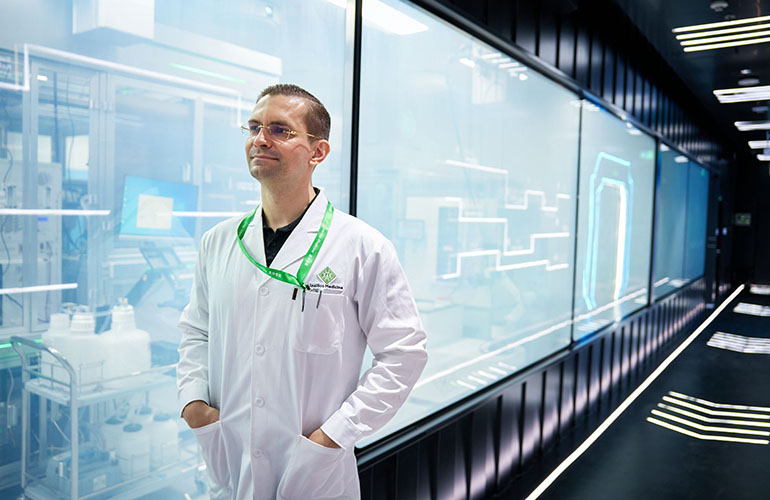
This is the AI-powered autonomous robotics lab called Life Star in Suzhou that we opened in January, which performs target discovery, compound screening, precision medicine development and translational research. [Insilico Medicine]
From its inception, the company has focused on aging and disease, and we wanted our lead program to be a “moonshot” drug – one that demonstrated beyond a doubt the validity of our AI drug discovery platform, Pharma.AI. So we were looking for a novel target for a chronic aging-based disease that would require a drug that met the most rigorous safety requirements — a drug that patients would need to take for the rest of their lives. Idiopathic pulmonary fibrosis met all those criteria — it is a devastating, chronic, and rare age-associated disease marked by progressive scarring of the lungs with poor prognosis and few treatment options.
Can you detail the results from the Phase I trials carried out in New Zealand and China?
Zhavoronkov: We enrolled healthy volunteers in the INS018_055 phase 1 clinical trial in New Zealand in February 2022 and in China in May 2022. Both of the clinical trials were completed in 2022. Top-line data from these trials indicated that the drug candidate has a favorable safety, tolerability, and pharmacokinetic profile. In Feb 2023 the drug received Orphan Drug Designation from the FDA, which supports the development of drugs for rare diseases like IP which affect fewer than 200,000 people in the U.S.
How is INS018_055 different from the other treatments available for IPF currently?
Zhavoronkov: Pirfenidone and nintedanib are the current treatments available to patients, which may provide some relief or slow the progress of the disease but do not reverse damage or stop the disease progression. They also have unpleasant side effects. There are very few options for people with this terrible condition, and the prognosis for those who are diagnosed is poor (most will die within two to five years of diagnosis). Our initial studies have indicated that INS018_055 can outperform the current standards of care with fewer side effects, and our drug may also work in combination with existing therapies to bring greater relief to patients.
In the Phase 2 clinical trials, what parameters will be evaluated and what is the proposed cohort structure?
Zhavoronkov: The study is a randomized, double-blind, placebo-controlled trial to assess the safety, tolerability, pharmacokinetics and preliminary efficacy of 12-week oral INS018_055 dosage in subjects with IPF divided into 4 parallel cohorts. To further evaluate the candidate in wider populations, the company plans to recruit 60 subjects with IPF at about 40 sites in both the U.S and China.
Can you elaborate on the Insilico AI-driven drug discovery process, the stages of target discovery, and the design and selection of this molecule?

Alex and Dr. Feng Ren, co-CEO and CSO of Insilico [Image courtesy of Insilico Medicine]
Chemistry42, our generative chemistry engine, was then applied to this novel target. We call this our “generative model zoo” – 500 predictive models are used, including transformer-based, GANs and genetic algorithms. All are pre-trained and they reward and punish each molecule that is dreamed up for how well it meets the necessary conditions – including hitting the target, metabolic stability and penetrability. We run this for 72 hours, and it requires huge computational resources to deploy. Ultimately we generate a select number to synthesize. In the case of INS018_055, we selected the 55th molecule synthesized out of 79 which showed promise in improving fibrosis and good safety profile in mouse models.
This system can imagine the perfect molecules for a given target that you would not be able to find in a chemical library.
What is the significance of this phase 2 trial for the AI drug discovery industry?

[Image courtesy of Insilico Medicine]
As this IPF drug advances, and has now entered trials with patients, we are in the critical final stages of demonstrating that this method of finding and developing new drugs works — and it can do the work faster, better, and cheaper than ever imagined. The success of this drug would mean that not only patients suffering from IPF, but from other diseases, can have new hope that AI can help speed new cures. We have 31 AI-designed drugs in our pipeline and three, including INS18_055, are in human clinical trials — 12 are at the preclinical candidate stage. Many of them are in the cancer space — including a USP1 inhibitor in clinical trials.
INS018_055 is a pan-fibrotic inhibitor. Will you be looking to expand into other types of fibrosis treatments?
Zhavoronkov: We’re very encouraged by the possibilities of this novel target which could provide an opportunity to treat not only IPF but larger fibrosis diseases, particularly kidney fibrosis. As we move the IPF program forward, we are demonstrating efficacy in IPF and will develop an additional set of biomarkers to understand the effect of the drug in fibrosis and monitor long term exposure.
But we recognize the very large need within kidney fibrosis. Chronic kidney disease affects some 37 million Americans and is of course highly age-associated, linked to diabetes, high blood pressure, and heart disease.
How does Insilico plan to continue advancing in the area of their AI-driven drug discovery process?
Zhavoronkov: We are continuing to incorporate the latest technological advances into our drug discovery platform to increase speed and accuracy. In January, we published a study using AlphaFold and Pharma.AI to accelerate the design of a novel drug for hepatocellular carcinoma, the most common primary type of liver cancer. It is the first successful application of AlphaFold to hit identification process in drug discovery. The study was led by a team of international team of researchers led by the University of Toronto’s Acceleration Consortium director Alán Aspuru-Guzik, Chemistry Nobel laureate Michael Levitt, and Insilico Medicine founder and CEO Alex Zhavoronkov and published in the journal Chemical Science. We see great potential for continued use of the AlphaFold predicted protein database with our platform. We’ve also added chat functionality into our target discovery engine, called ChatPandaGPT, allowing users more seamless interaction with the knowledge graph that connects diseases and possible targets.
And in another international collaboration, we also recently published a study combining quantum computing with generative chemistry to generate new molecules. It’s the first step in what we believe will be the future of drug discovery and was published in the Journal of Chemical Information and Modeling.
Filed Under: clinical trials, Data science, Drug Discovery, Industry 4.0, machine learning and AI, Pulmonology