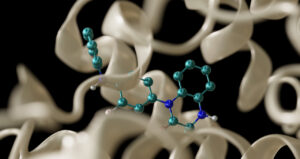
The most recent NVIDIA BioNeMo foundation models can help drug discovery groups design drug molecules virtually. [Credit: NVIDIA]
“For the first time in history, we can represent the world of biology and chemistry in a computer, making computer-aided drug discovery possible,” said Kimberly Powell, NVIDIA VP of healthcare, in a press briefing. “By helping healthcare companies easily build and manage AI solutions, we’re enabling them to harness the full power and potential of generative AI.”
The latest additions to BioNeMo, announced today at NVIDIA GTC, include new foundation models for key tasks such as analyzing DNA sequences, predicting protein structures and determining cell function based on RNA data. These models are now available as simple-to-deploy microservices through NVIDIA NIM (NVIDIA Inference Microservices), a set of cloud-native microservices designed to simplify the deployment of generative AI models in production environments. NIMs support the integration of AI processes into applications using industry-standard APIs while abstracting away the complexities of AI model development.
New foundation models for genomics and protein design
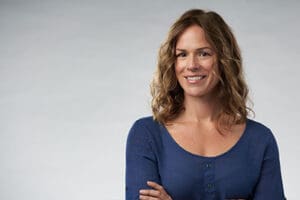
Kimberly Powell
Among the new foundation models available in BioNeMo is DNABERT, the platform’s first genomics model. The pre-trained bidirectional encoder representation model supports exploring DNA sequences in the genome. Trained on copious amounts of DNA sequence data, DNABERT can predict the function of specific regions of the genome, analyze the effects of gene mutations and variants. NVIDIA says the tool can help researchers better understand the genetic basis of diseases and identify potential therapeutic targets.
Another upcoming addition to BioNeMo is scBERT, a model trained on single-cell RNA sequencing data. This model enables users to predict the effects of gene knockouts and identify specific cell types, such as neurons, blood cells or muscle cells. scBERT can aid in the development of targeted therapies and precision medicine approaches.
EquiDock, a third new model, joins BioNeMo’s portfolio of tools for predicting the 3D structure of protein interactions. EquiDock can help researchers identify the most promising drug-protein combinations, streamlining the drug discovery process.
Microservices and flexible deployment options
The containerized AI microservices available through NVIDIA NIM features more than two dozen healthcare models, including DiffDock for predicting the 3D structure of drug candidates and their protein targets, ESMFold (originally developed at Meta) for predicting protein structure based on amino acid sequences, and MolMIM for generating drug candidates optimized for user-defined properties.
Developers can access these production-grade NIM microservices through NVIDIA AI Enterprise using NVIDIA-Certified Systems on-premises, as well as through leading cloud marketplaces like AWS, Google Cloud, Microsoft Azure, and Oracle Cloud Infrastructure. NVIDIA says the flexibility will make it easier for pharmaceutical and biotech companies to seamlessly integrate generative AI into their existing drug discovery workflows, regardless of IT infrastructure.
Exploring larger drug spaces
By integrating these AI capabilities directly into their discovery workflows, drug hunters can tap generative AI to virtually explore larger universes of potential drug candidates, better understand mechanisms of action, and crunch the time and cost associated with physical experiments. Companies such as Cadence, which is integrating BioNeMo microservices into its Orion molecular simulation platform, are already deploying the technology to accelerate their pharmaceutical clients’ efforts.
Another firm using the technology is Iambic, which has demonstrated the power of BioNeMo by slashing drug development timelines for IAM1363, a drug candidate that went from program launch to clinical studies in fewer than 24 months.
“Generative AI is opening up healthcare to be one of the largest technology industries,” Powell said. “The hope is that this incredibly increases the demand because generative AI is a breakthrough capability across all of these different domains, from digital surgery to drug discovery and digital biology to digital health.”
Filed Under: Data science, Genomics/Proteomics, machine learning and AI, Oncology, Uncategorized