
[Adobe Stock]
The sorcerer’s apprentice: Enthusiasm and the need for mastery
Much like the eager apprentice in Disney’s animated film “Fantasia,” which itself is inspired by Goethe’s “Der Zauberlehrling”(“Sorcerer’s Apprentice“), many in the clinical research field seem eager to unleash the power of AI even before fully grasping the strategic investment required for mastery. It’s a familiar pattern for emerging technologies — early hype outpacing practical adoption. While 80% are either exploring or planning implementation, the report reveals that only 7% of respondents report successfully integrating AI/ML across applications.
Marrying big dreams with strategic steps
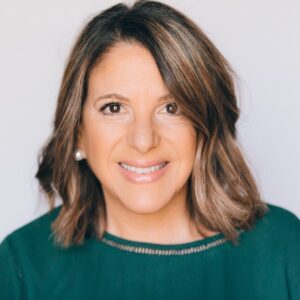
Diane Lacroix
Some organizations’ enthusiasm to deploy AI/ML can translate into projects that are “too wide,” said Diane Lacroix, vice president, clinical data management at eClinical Solutions. It’s often better to focus on smaller components of applying AI where access to high-quality data exists. “You can have an AI/ML strategy, but if you don’t have access to the high quality data that you need to develop those models, that’s the wrong place to start,” Lacroix said.
Clinical trials: Can data management keep pace with the data explosion?
The vast amounts of data generated in clinical trials are creating bottlenecks that existing systems struggle to manage. Lacroix highlights this by noting challenges with bringing in real-world data, sensor data, and other streams generated by decentralized clinical trials (DCTs). Traditional electronic data capture (EDC) systems often lack the necessary flexibility and interoperability to integrate and standardize these diverse data sources.
This struggle to unleash the full potential of available data isn’t unique. In the Industry Outlook 2024 report, Emily Pereira, director, clinical data management from bluebird bio mentions, “We’re seeing a lot of external data sources that are bringing in data in so many ways. We’re trying to house that data externally from EDC, which is a good thing, but the question becomes, ‘How do you bring all of this data in and make it useful for reporting purposes?’”
While some industry observers have welcomed the emergence of a post-EDC era, Lacroix opined, “I think EDC is going to be around for a while.” Instead of quickly disappearing, the technology is poised to evolve to be more interoperable with decentralized trial technologies, she added. Such a transformation is necessary as DCTs generate real-world data, sensor data, and other valuable streams that might not fit neatly into existing EDC frameworks.
External data integration stalls: What’s holding back adoption?
The integration of external data sources in clinical trials has recently plateaued. Recent survey data reveals that the largest segment of respondents used between 6 and 10 external data sources, followed by those using 3 to 5 sources. “When we did the survey last year, the respondents reported that the average number of data sources was somewhere around six to 10,” Lacroix said.
The numbers were somewhat lower in the recent survey. The differences could be a result of changes in audience or the phase and design of trials. But another factor that could be at play is a cooling phase in adoption of decentralized clinical trials, wearables and sensors in clinical trial settings. In the pandemic, “we were forced to explore different ways of collecting data using different technologies,” Lacroix said.
Still, the numbers are up when taking a broader view. “A decade ago, we were maybe collecting two or three external data sources,” Lacroix recalls. “It was very site-centric, EDC-centric.” Transforming our approaches to manage this complexity without extending cycle times represents an ambitious undertaking.
Balancing present and future needs
Lacroix highlights the balance between investing in long-term advances such as AI/ML-enabled processes and addressing the immediate need to improve cycle times — a challenge she describes as “always at the top of the conversation.” This emphasis on cycle time resonates deeply with both sponsors and companies like eClinical. “We want to optimize technology, people, and processes to continue to decrease cycle time,” Lacroix states.
Additional concerns include pressures like diminishing R&D returns and patient recruitment hurdles. To address these challenges, eClinical is deploying a suite of advanced technologies and specialized services to streamline and accelerate trial processes. This strategy includes AI-driven data review, automated EDC builds, and a focus on condensing the often-lengthy period between Last Patient Last Visit (LPLV) and database lock. This approach has yielded impressive results, enabling eClinical to reduce the LPLV to database lock timeframe to 2-4 weeks, significantly outperforming the industry average of over 6 weeks.
Promise and the path to AI adoption in clinical trials
When asked if there were any surprising points of the survey results, Lacroix points to the AI/ML results, noting that the technologies are poised to have a significant impact in the next 12 months, but “most of our respondents haven’t really started implementing. We all recognize there’s a definite gap between the promise and the reality,” she said. “I was a bit surprised to see that risk-based strategies were quite low on the list, around 13%. Considering FDA’s guidance on the subject, asking the industry to implement risk-based strategies the findings were “surprising,” Lacroix said.
“We often hear that risk-based strategies are better suited for phase 3 or phase 4. But the guidance doesn’t specify that we shouldn’t use risk-based approaches in phase 1 and phase 2,” Lacroix clarified. “A lot of the bigger sponsors are focusing on more risk-based approaches,” she said. However, many smaller players still operate under a different mindset.
AI in clinical trials: From data management novice to master AI clinical trials
Lacroix emphasizes the need for a broader shift across the industry. While risk-based approaches are often implemented at a quality management level and in clinical operations, data management is still asked to validate data at 100%. Data management strategies must adapt to accommodate the influx of clinical data. “It’s impossible to clean all of it,” Lacroix said.
Here, ML technologies can play a role in sifting through the vast data volumes. “As opposed to sitting down to look at lab data and looking at thousands and thousands of lab records, the models are running through those. They’re surfacing outliers,” Lacroix said. Such models can explore why some lab values stand out from similar tests for other patients in the study. “It’s accelerating our time to data insights,” she said.
Using tools to enhance efficiency doesn’t necessarily entail treating all data equally. “Maybe we use artificial intelligence to look at your non-critical data, right?” Lacroix said. A risk-based approach doesn’t mean that non-critical data is ignored. It simply means the analysis might be tailored to its level of importance, while still preserving it for potential data mining insights down the line. This opens up possibilities for automation and even further use of AI to pinpoint safety issues or anything that poses a risk to the trial’s success.
“We spend a lot of time sifting through data to find the needle in the haystack versus being presented with the needle in the haystack,” Lacroix added. “That’s really what AI/ML is doing for us with the models that we’ve been deploying.”
Filed Under: Uncategorized