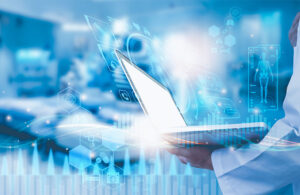
[Adobe Stock]
Certainly, AI is hyped, but so are potential profits of potentially AI-optimized drugs. The exploding volumes of scientific data highlight a shift often overlooked: what does “inventor” even mean when human brilliance relies on AI and vast datasets no single person can comprehend? This future depends in part on connecting the dots between data experts, lab scientists with domain knowledge, and the machine learning systems capable of pattern recognition humans can’t even fathom. But the crux isn’t simply generating more data, and making it a shared, dynamic force fueling breakthrough discoveries — a force deeply integrated with computation and human expertise.
Breaking through the data bottleneck
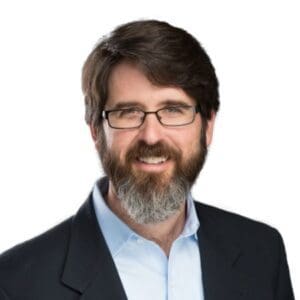
Michael Connell
While technologies like generative AI evolve rapidly, if data can’t keep pace, progress is limited. Today, as Enthought COO Mike Connell observes, many companies inadvertently cordon off key research findings in PDFs, PowerPoints, and Word documents. If they later decide to mine them for insights, “they have to go and search all these PowerPoints, for instance, for images,” Connell said. In other cases, they might sift through millions of PDFs with pictures of problems and known solutions. “Finding specific information in these formats isn’t possible through keyword search,” he noted.
Further complicating the picture, individual scientists might store research findings locally on laptops or in cloud storage accessible only to a small team. This decentralized approach makes it nearly impossible to establish a single source of truth, hindering knowledge sharing and collaboration. “This is not treating data as a product. It’s quite the opposite,” Connell said.
This reality often leaves researchers’ peers — or ML systems — unable to mine previously unearthed scientific insights. As research becomes ever more specialized, this inefficient hunt risks burying vital connections to fuel future scientific breakthroughs.
Skynet as lab partner: Creating a superorganism with self-improving AI and human intellect
AI’s potential in a laboratory setting extends beyond simply supporting scientists — it empowers a shift where researchers become “commanders.” In military operations, the commander’s intent is a concise statement that describes the desired endstate and purpose of the operation.
With access to the right datasets, AI systems with iterative learning could rapidly sift through past studies, spot unexpected links, and, just as Connell suggests, even propose entirely novel experiments based on a “commander’s intent.” This independence and iterative problem-solving allow the AI to analyze data, formulate strategies, and run experiments entirely driven by the directive it’s been given. Taking matters a step further, Alphabet’s DeepMind has embedded PaLM-E in robotic frameworks. Ultimately, human experts remain “commanders” — issuing objectives, validating AI discoveries, and steering its capabilities towards impactful breakthroughs.
Imagine the traditional approach of humans working step by step to devise a protocol for, say, a new leukemia treatment that targets the faulty blood cell production without the immune-suppressing side effects of chemotherapy drugs. Now, envision this: “Letting the machine find it for you through a combination of searching the data that’s out there and then running its own experiments,” Connell said.
Using AI to further AI
While the ultimate goal isn’t to hand over control to AI, AI is evolving so rapidly that it becomes increasingly necessary to use AI to make sense of, and align, other AI systems. “That’s the only way to do it, right?” Connell said. “So they’re training these other LLMs and other networks to analyze those networks. And so it’s Skynet, right?”
The Skynet dimension (or any trope involving a fictional AI gone rogue), however, can also arouse fear — and that emotion may blind some to the tool’s real-world potential. When discussing AI capable of such independence, pop culture tropes fuel anxieties, ranging from a threat to livelihood to widespread automation of tasks in bench science, data analysis, and even business decisions. On the other hand, tech optimists tend to have more of a fear of missing out on future possibilities if they delay adopting, or miss out on breakthroughs their competitors discover first through AI-accelerated research.
AI agents: Scientists’ force multipliers?
The vision of an AI-powered self-driving lab is a distinct relatively near-term possibility within drug discovery “where AI independently learns and acquires knowledge,” as a recent Nature article highlighted.
AI agents, programmed to tackle the complexities of protein analysis and compound design, hold the potential to be scientists’ force multipliers. Imagine agents capable of streamlining workflows, freeing up researchers to focus on the complexities of target identification and hypothesis generation. Or, envision an agent with the capacity for “autotelic learning” — where it sets its own goals within the scientific domain and uncovers breakthroughs that might slip past human scientists, as the aforementioned Nature article suggests.
Standing on AI shoulders
In a collaborative model, the AI isn’t simply a tool automating existing tasks; it increasingly becomes a source for human knowledge — a new sort of summit to stand on. When asked what allowed him to break new intellectual ground, Isaac Newton quipped, “If I have seen further than others, it is by standing upon the shoulders of giants.” The same principle often holds for AI agents whose knowledge is based on the tireless work of human researchers. This foundation can become a launchpad for further discovery where well-designed AI systems synthesize information at a scale inconceivable to individual minds. As Pierre-Yves Oudeyer stated in Nature, “agents could form the basis of embodied intelligence, which might eventually lead to self-driving labs where AI independently learns and acquires knowledge.”
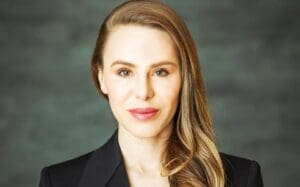
Dr. Michelle Longmire
In a recent episode of AI Meets Life Sci, Dr. Michelle Longmire, CEO of Medable, painted a sobering picture: “Right now, at the current pace, there are approximately 10,000 uncured or poorly suboptimally treated human diseases, it’s going to take us about 200 years to create treatments for all of those different conditions.” However, she envisioned a radical shift: “Imagine if we could 10x that… then that timeline goes from 200 years to 20 years, something potentially feasible in most of our lifetimes.”
Making such a vision a reality won’t be possible with siloed AI systems, fragmented data or isolated researchers storing valuable findings in isolated spreadsheets or PowerPoints. “There’s an enormous amount of latent value out there that’s inaccessible,” Connell said. It’s hard to fathom the value hidden in obscure patents, past failed research, even overlooked older academic work.
Unshackling untapped data fuel
While much has been made of the exploding volumes of data in medical and scientific research, much data is frequently underutilized. If we don’t treat data as “a valuable resource… as a data product,” as Connell urged, neither human researchers nor AI can leverage it. Instead of fearing the emergence of a real-world Skynet, a more productive strategy is to step up and play an active role in deploying and aligning AI systems with altruistic values.
This shift wouldn’t just transform labs, but our approach to knowledge itself. “Imagine a drug discovery lab where the traditional focus on individual researchers is replaced by interconnected agents,” Connell said. We could break down tasks currently carried out by humans, allowing the lab to become something fundamentally different — a kind of advanced “computing box,” as Connell put it. Yes, it analyzes data, but it also acts upon the physical world, creating and testing new compounds in a sort of closed loop. With AI tireless and free from some of the pitfalls inherent to human work, this shift lets us rethink science — and the creation of research literature and data — at a higher level. Perhaps instead of focusing on specific steps, entire experiments could be designed, refined, and shared seamlessly with other labs worldwide.
It’s not overlord AI we should fear — it’s human inertia
Skynet was self-contained, seeking knowledge independently. The aim isn’t to base tangible AI plans on science fiction tropes, but to build something fundamentally collaborative and carefully aligned to altruistic aims. Maybe the fear of Skynet isn’t the AI getting too smart, but us becoming intellectually inert as it does our grunt work. Avoiding this scenario requires laying the foundation for a reality in which data, computing, humans and algorithms are aligned in a way that is mutually beneficial and self-correcting. This is Skynet, reimagined.
Filed Under: Biotech, Data science, machine learning and AI