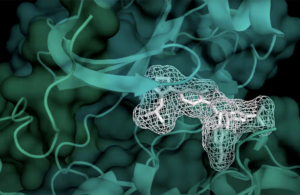
Virtual screening of a kinase with AtomNet technology [Atomwise]
In the virtual high-throughput screening (HTS) study, AtomNet analyzed 318 targets in collaboration with more than 250 academic labs across 30 countries. The paper had more than 600 authors. The study concluded that AI could be a viable alternative to traditional HTS methods. “To my knowledge, this is by far the largest and most diverse demonstration of an AI-driven drug discovery platform,” said Abraham Heifets, CEO of Atomwise. “I think that’s critical for the scientific community to gain confidence in adopting these approaches broadly.
Atomwise sees neural nets enabling ‘a generational shift’ in drug discovery
Heifets said the results provide an alternative to HTS, “a tried and true technology” with decades of history in drug discovery. “What we’re talking about here is potentially a generational shift, which is exciting,” Heifets said. “As technology evolves and the kinds of targets we’re looking at get harder, you want to ask: Are there new ways of extrapolating and finding new parts of chemical space? That’s something we generally want from AI.”Heifets emphasizes that the conversation around AI in drug discovery should focus on its ability to deliver first-in-class or best-in-class medicines, rather than simply speeding up existing processes. “What I’m saying is we should have a different conversation: can we do better than we’ve been doing, or can we do different than we’ve ever done? That’s a different conversation.”
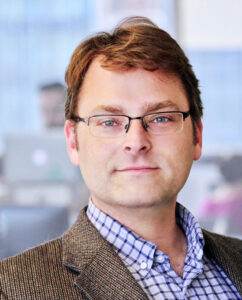
Abraham Heifets, Ph.D.
At the heart of Atomwise’s approach is convolutional neural networks. “You have used them if you’ve ever talked to Siri or Alexa, if you’ve ever unlocked your iPhone with your face,” Heifets said. “It’s arguably still our species best technology for image recognition, speech recognition.” Atomwise claims to be the first to apply the technique to molecular recognition.
Expanding chemical space with AtomNet
AtomNet’s ability to search a chemical library of over 15 quadrillion synthesizable compounds offers unprecedented potential for drug discovery. “This gives us the promise of testing chemical space way outside where we’ve ever had access to,” Heifets said.
The study’s results demonstrate AtomNet’s potential to identify novel chemical matter for challenging targets, with implications for developing first-in-class and best-in-class therapies across major therapeutic areas. “Across those projects, we achieved about a 74% success rate when our partners said, ‘Hey, that worked.’ That’s actually higher than what people report in the literature for high throughput screening, which is about 50%. That’s exciting,” he said. The technology didn’t require precise target data or X-ray crystallography structures. “For the targets where hits were identified, we discovered an average of seven distinct bioactive compounds,” Heifets said. “That gives medicinal chemists multiple different paths to take forward.”
While the study’s results are promising, Heifets emphasizes the need for preclinical data and clinical validation. “At the end of the day, in pharma, what matters is the behavior of these drugs in the human animal. We do a lot of testing in the preclinical phase, and that’s what we’ve been focused on,” he said.
Changing the standard workflow in drug discovery
Heifets draws an analogy to the adoption of electricity in manufacturing: “In every other major industry, you simulate 1,000 different wings before you build one, whereas in pharma, you hand-whittle every prototype and then test the prototypes physically. If we time-traveled someone from 1900, they would recognize test tubes. That’s interesting.”He believes that AI has the potential to change the standard workflow in drug discovery. “The majority of new chemical space and new scaffolds have come from one technique that’s the standard workhorse of high throughput screening. To change that, to change the standard workflow, you would need to show that a new technique can work on whatever protein you throw at it, with or without data, with or without structural enablement, etc.”
Atomwise’s TYK2 inhibitor serves as an example of AI exploring new chemical space. “For TYK2, we think it’s a good example of what happens when AI explores different parts of chemical space. […] We’ve got all this structure over here that deucravacitinib lacks, and then this amide that Bristol Myers scientists write about as being critical to selectivity is absent in our molecule. We took a different strategy for selectivity.”
Filed Under: Drug Discovery, machine learning and AI