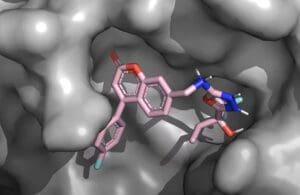
[Image courtesy of Nvidia]
To date, NVIDIA is offering about two dozen new healthcare microservices with more to follow.
NIMs intend to help scale AI deployment across infrastructures
NIMs, which NVIDIA announced on March 18 at its GTC conference, provide a streamlined path for deploying AI models across infrastructures while maintaining data security and control. Powell also pointed out that the approach prioritizes ease of deployment and cost. The NIMs offerings stem from the debut of NVIDIA’s BioNeMo, a cloud service that aims to accelerate drug discovery research.
Beyond flexibility, Powell emphasizes the importance of NIMs accessibility: NVIDIA wanted to make the tools accessible – both in terms of ease of deployment and cost — so scientific teams can focus on productivity rather than learning how to use new tools. “These scientific teams can do science the way they’ve been doing,” she said. “And in parallel, they can learn how AI is doing it.”
The advantages of microservices architecture
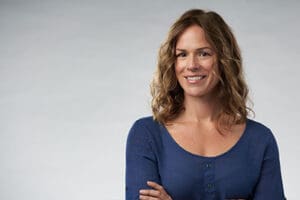
Kimberly Powell
Powell emphasizes the importance of microservices architecture for the success of NIMs. “Microservices were a major driver in the rise of cloud technology,” she said. A microservice is really just a mini-application that has all of its dependencies packaged up in one place with a defined set of inputs and outputs. To illustrate how this architecture can accelerate deployment, she explains the complexity involved in developing AlphaFold 2 from scratch and putting it into production: “There are many things you would have to do: first, identify the most accurate AlphaFold 2 model; then figure out how to run it efficiently, which is costly; optimize it if needed; containerize it; design APIs; and finally, manage ongoing security and maintenance, as required for production software.” Microservices streamline and simplify this complex deployment process, providing a pre-packaged solution that abstracts away infrastructure challenges.
Early biopharma adopters showcase the potential of AI-based NVIDIA microservices
Powell highlights early real-world wins involving microservices within the biopharma industry. She points to NVIDIA partner, Amgen, which has used similar technology to compress early development, reducing, for instance, a timeline that may have taken two years in the past to just nine months. “Additionally, the likelihood of those candidates successfully reaching the clinic has increased from 50% to 90%,” she said.
Additionally, in a session at NVIDIA’s GTC event, Aviv Regev, head of Genentech Research and Early Development described an AI system that led to a 50x improved hit rate of small molecule potency over traditional medicinal chemist methods.
The adoption of AI technologies will not require pharma companies to transform into technology companies per se to keep up in a rapidly evolving technological landscape. Instead, they can incorporate these tools into their existing workflows at different levels of intensity.
“I believe that’s going to be a light bulb for them to say, why wouldn’t we have this running around rapidly and accessible inside of our R&D teams as another set of tools in the work that we do?” Powell said.
While the potential of generative AI in the biopharma industry is significant, its success will depend on the ability of companies to effectively integrate these new technologies into their existing workflows and navigate the challenges that come with adoption. Engineers and researchers also must work together to envision the future of scientific research. The Royal Society, for instance, has described an “AI revolution in scientific research” thanks to the potential of AI tools to extract insights from large data sets, redefine scientific models and uncover previously unknown relationships. “Some luminaries,” Powell said, predict that AI tools “could redefine the scientific method itself.”
Filed Under: Drug Discovery, machine learning and AI