Founded in 2017, VeriSIM Life (San Francisco) has a quest to use biosimulation technology to streamline drug discovery and development. The company’s BIOiSIM virtual drug development engine helps pharma and biotech companies optimize R&D spending.
The company’s founder and CEO, Dr. Jo Varshney, also wants to help reduce the need for animal testing in drug development. The industry’s “reliance on animal testing has been ridiculously bad for the industry, if not horrible in terms of time and costs,” said Varshney, a veterinarian.
Varshney sees the Senate’s unanimous passage of the FDA Modernization Act 2.0 as a good omen. The legislation, if signed into law, would reduce animal testing in the coming years.
Other factors bode well for reducing the industry’s reliance on animal testing. First, computational resources continue to become less expensive and more powerful, making AI drug development models increasingly accurate. Second, there is growing traction for the 3Rs, a decades-old term referring to the “replacement,” “reduction” and “refinement” of animal testing. International regulators and Big Pharma have endorsed the 3Rs.
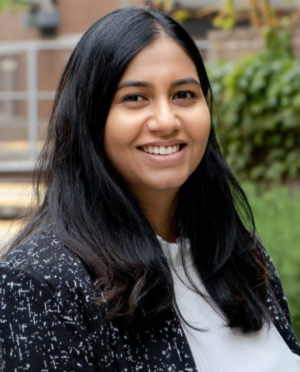
Dr. Jo Varshney
Varshney credits her interest in drug development to her father, who began working in drug development when she was two. “The animal models didn’t make sense to me then, and they still don’t make sense now, considering how much we randomly test things in animals,” she said.
In addition to her veterinary background, Varshney holds a Ph.D. in comparative oncology and genomics, and a master’s in translational pathobiology and bioinformatics. “I’m not one of those founders that dropped out of school,” she said.
It is fitting that VeriSIM has taken a multidisciplinary approach when developing its BIOiSIM platform, which offers inform an index to capture the translatability from animal models to humans. “It’s very similar to a FICO score. When you get that score, you know how your financial health is,” Varshney said. “Similarly, with the translational index score, we can work with clients and tell them the score and recommend various methods to either reduce, refine or replace animal testing.”
The machine learning models also help drug developers plan on what “potential blind alleys” they may encounter in future drug development efforts for a chosen molecule while reducing risk, Varshney said.
VeriSIM Life envisions BIOiSIM as an ultimately autonomous system that can inform drug development strategy as candidates progress through the pipeline. For example, before a drug candidate enters human trials, BIOiSIM can help drug developers identify the best non-clinical or preclinical system to help predict its response in humans. The system is an alternative to “blindly going into random testing in different animals,” Varshney said.
While researchers have spent billions of dollars creating drugs that can cure diseases in mice, curing diseases in humans tends to remain a thornier endeavor. Inaccurate animal models are partly to blame. The use of animals in drug development is “almost like a religious belief,” Varney said. “People have been trained to think about animal systems for more than 50 years in every stage of drug development.”
The adoption of AI in drug development could be similar to that of self-driving car technology. However, while the technology is quickly advancing, full-scale adoption will require a paradigm shift.
“Honestly, if you ask scientists in this field, no one wants to do animal testing because it’s brutal,” Varshney said. “But you have to do it now to show proof of concept and such.”
VeriSIM Life plans to take a more evolutionary approach than a revolutionary one in working with clients. “If you have done animal experiments, you can input those into our system, and we’ll tell you which animal model is best to move forward,” she said. “If you have not done animal testing, we can tell you which animal to choose.”
The data informing the BIOiSIM’s decision-making comes from a range of sources. The company pulls from internal and publicly-available data. VeriSIM Life also has several data partnerships specific to disease areas. “So with all that data accumulation, we test our system constantly on different outliers and in different ways,” Varshney said. “We test to see how well our system compares to an animal outcome, which is a real metric, versus how well our system compares to a non-animal outcome.” The company is constantly working to assess how accurately BIOiSIm can forecast risks for a given asset to “make it more accurate and efficient in terms of less reliance on external data,” Varshney said.
Filed Under: clinical trials, Drug Discovery, machine learning and AI, Women in Pharma and Biotech